Industry Insights
Ideas to Inspire
- Forbes
- Jessica Hawthorne-Castro
Leadership transitions signal change—and opportunity. But how do brands navigate founder exits and new leadership with PR that supports credibility, continuity, and momentum? In Forbes Agency Council latest article, Jessica Hawthorne-Castro shares her top strategy to ensure smooth transitions.
- Forbes
- Jessica Hawthorne-Castro
As AI shopping assistants become a bigger part of the retail experience, how can brands ensure they’re communicating effectively—without losing the human touch? In Forbes Agency Council latest article, Jessica Hawthorne-Castro shares her tips for optimizing AI-powered retail interactions. From using brand-aligned messaging and emotional cues to prioritizing transparency and real-time learning, the insights aim to help retail brands build trust and drive conversions in the age of AI.
- Forbes
- Jessica Hawthorne-Castro
Starting a new role remotely can feel like being dropped into a new world without a map. For agency leaders, helping new hires find their footing—fast—isn’t just good onboarding, it’s good business. In Forbes Agency Council latest article, Jessica Hawthorne-Castro shares her tips to help remote team members ramp up more quickly and feel connected from day one. If you're leading a hybrid or remote team, it's well worth the read.
- The Business Journals
- Jessica Hawthorne-Castro
Discover how companies can better support employee well-being through strategic benefits, flexible policies and a culture of care. In Business Journals Leadership Trust latest article, Jessica Hawthorne-Castro offers a practical framework leaders can follow to support their employees.
- Forbes
- Jessica Hawthorne-Castro
Live shopping is no longer a trend, it’s a transformation. From real-time engagement to instant conversion, live commerce is redefining how brands connect with consumers. In Forbes Agency Council latest article, Jessica Hawthorne-Castro provides insights into why live shopping is driving serious e-commerce growth in 2025. If you're in marketing, retail or digital strategy, it's worth the read.
- The Business Journals
- Jessica Hawthorne-Castro
Promoting from within can strengthen company culture, boost morale and improve retention—but identifying the right internal leaders takes strategy and clarity. In Business Journals Leadership Trust latest article, Jessica Hawthorne-Castro offers a practical framework for screening internal leadership candidates effectively. Great insights for any organization focused on long-term growth and talent development.
- Forbes
- Jessica Hawthorne-Castro
In today’s fast-moving digital world, a brand’s reputation can shift in an instant. Having a crisis communication plan in place isn’t optional—it’s essential. In Forbes Agency Council latest article, Jessica Hawthorne-Castro outlines her strategy that can help businesses build a strong, strategic crisis comms plan before they need it. Whether you're in PR, marketing or leadership, this is a must-read for staying prepared and protecting brand trust.
- The Business Journals
- Jessica Hawthorne-Castro
Empowerment isn’t about stepping back—it’s about stepping aside with purpose. In Business Journals Leadership Trust latest article, Jessica Hawthorne-Castro offers a thoughtful strategy on how leaders can strike the right balance between offering guidance and granting autonomy.
- The Business Journals
- Jessica Hawthorne-Castro
Client retention isn’t just about great service—it’s about building trust, delivering value and avoiding missteps that can quietly erode relationships over time. In Business Journals Leadership Trust latest article, Jessica Hawthorne-Castro highlights what critical mistake B2B companies often make when it comes to keeping clients engaged and loyal.
- Forbes
- Jessica Hawthorne-Castro
Staying ahead of ever-evolving social media trends is no easy feat—but it’s essential for brands and agencies looking to stay relevant and drive real results. In Forbes Agency Council latest article, Jessica Hawthorne-Castro highlights how agencies are already leveraging the latest platform updates to connect more meaningfully with audiences.
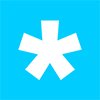
Resources
Hawthorne has been a leader and pioneer in Brand Response Advertising and Direct Response Marketing for 35 years.
Our rich history of measuring audience response and applying data-driven attribution and analytics to optimize campaigns provides us with an unparalleled depth and breadth of experience. Some of that experience is shared below in this Resources section.
Drop us a note to explore how
You can future proof your
brand and business